The Jigsaw Method in 12th-Grade Physics Classes Impact of the Group’s Ability Composition on Academic Performance
Roland Berger1, *, Martin Hänze2
1Physics Education Group, Department of Physics, University of Osnabrück, Osnabrück, Germany
2Institute of Psychology, University of Kassel, Kassel, Germany
Email address

(R. Berger)
*Corresponding author
Citation
Roland Berger, Martin Hänze. The Jigsaw Method in 12th-Grade Physics Classes Impact of the Group’s Ability Composition on Academic Performance. International Journal of Modern Education Research. Vol. 3, No. 5, 2016, pp. 28-36.
Abstract
Two hundred thirty-six students in 12th-grade physics classes took part in a quasi-experimental study comparing homogeneous and heterogeneous grouping in a jigsaw classroom. Students were assigned to expert groups according to their prior topic knowledge. Students showed higher intrinsic motivation, activated deeper level processing strategies, and performed better on their expert topic when working in homogeneous groups. Self-reported quality of communication was identified as a partial mediator between the composition of the expert group and academic performance.
Keywords
Cooperative Learning, Jigsaw Classroom, Group Composition, Physics
1. Introduction
An impressive body of research shows that cooperative learning methods can foster positive outcomes, ranging from increased motivation to improved academic achievement [1]. An important factor for fruitful cooperation is the composition of the group [2]. Students can be assigned to a group by self-selection, random selection or other criteria, e.g., a student’s interest in the topic or gender. From a cognitive perspective, assignment by ability or prior knowledge is of great interest. One main research result is that neither form of ability grouping is uniformly superior for promoting the achievement of all students [3]. One striking disadvantage of homogeneous grouping is that it has a detrimental impact on the performance of low-ability students [4].
In order to address this problem, we have conducted a study based on the jigsaw learning technique, a widely used method that was first proposed by Aronson in the 1970s [5] [6]. This form of group work involves students switching between different groups. Students first get together with others who have been assigned the same subtopic in the so called “expert groups Together they research and discuss their subtopic, and clear up questions. Subsequently, these expert groups break up and the students recombine with experts on other subtopics to form “jigsaw groups Each student in each group then teaches the rest of the group about his or her expert subtopic. In this way the whole topic is covered.
The jigsaw method combines two key elements that must be present for students to be successful in a cooperative learning environment. These elements are individual accountability and positive interdependence [7]: due to task-specialisation, each member of a group is accountable for a unique part of the activity; students in the jigsaw groups are then dependent on the knowledge of their experts. Furthermore, students in the expert groups are aware that they are expected to teach their peers in the jigsaw groups (“teaching expectancy.
There has been little research on the most effective group composition in science education. Furthermore, as far as we can see, none of the studies in the literature has focused on group composition in cooperative expert models such as the jigsaw learning technique.
This cooperative method should be of particular interest in light of role theory [8] [9]: Student experts are required to teach their jigsaw groups, a role that is associated with competence, prestige, and authority. A successful role enactment depends on whether a student perceives him- or herself in a way that is consistent with the expectations of the role. Thus, role enactment in the expert group is less likely if the perceived competence of a group member is incompatible with the subsequent requirement to teach the jigsaw group. It is well known from social psychology that one’s self-perception relies to a large extent upon the responses of others [10]. The discrepancy between self-concept and the demand to teach might thus be compounded when low-ability students are confronted with high-performing, “real” experts when working in a heterogeneous group that contains high-ability students. Under these circumstances, no congruence between the characteristics of self-concept of ability and role requirements can be established. Thus it is questionable whether students with low prior knowledge will be able to accept their role as an expert in an expert group that contains a wide range of abilities. If students expect to fail when they go on to teach their jigsaw groups, this might be detrimental for their experience of competence as well as their self-concept of ability.
This paper focuses on the question of whether expert groups of homogeneous ability in the jigsaw classroom set-up will benefit all students. Learners with teaching expectancy should be more motivated in understanding the material at a deeper level [11]. Thus, teaching expectancy should induce more interaction that involves all students.
1.1. Perspectives on Cooperative Learning
The existing body of empirical studies converge on the notion that giving and receiving explanations and help are productive interactions that are essential for the success of working cooperatively ([12] [13]; in the realm of science education cf. [14]).
From a theoretical point of view, there is no unifying approach that could consistently explain all the possible outcomes of cooperative learning. In the following, short descriptions of the perspectives that are relevant for our study will be given (cf. e.g. [1]). Vygotsky stated that an individual’s cognitive development stems from social interaction. According to his sociocultural approach, the interdependence of social and individual processes leads to co-construction of knowledge that is supported by operating in a learner’s zone of proximal development, that is, “the distance between the actual developmental level as determined by independent problem solving and the level of potential development as determined through problem solving under adult guidance or in collaboration with more capable peers.([15], p. 86).
From the perspective in the tradition of Piaget, discussions in cooperative learning settings confront learners with differing or controversial views, thus stimulating an adaptation of the cognitive structure of group members until equilibrium is reached. Thus, cooperation is fruitful, since students can help each other to enhance their individual levels of competence.
From the viewpoint of cognitive elaboration, learners must try to adapt their knowledge structure in order to integrate new information. High quality of interaction (e.g., asking questions; reasoning about the subject matter) in cooperation settings promotes fruitful learning by activation of strategies for deeper level processing of information [16] [17]. Following Springer, Stanne and Donovan [18], one of the most effective means of elaboration is explaining the material to others. This should help the explainer to reorganize the information in new ways, which may help the explainer to understand the learning material better. Furthermore, in this way the tutor might detect knowledge gaps in his or her understanding [19].
From the perspective of social cohesion, students will engage in the task and help each other because they identify with the group. In order to foster cohesiveness within a group, Cohen [20] emphasized the relevance of setting a meaningful and challenging task. Furthermore, she underpinned that the success of cooperation strongly depends on the quality of interaction within the group.
1.2. Research on Group Ability Composition
Several major meta-analyses on the effects of within-class grouping on student achievement have been conducted. Lou et al. [4] included most of the studies reviewed earlier. Aggregated across 12 studies that focused on the ranges of ability within small groups, the authors found that groups with homogeneous ability were better than those of heterogeneous ability in promoting academic achievement, although the effect was small (d = 0.12)1. The results varied considerably across the studies, indicating the influence of moderating variables. Lou et al. identified the subject being taught as a significant moderator of the effect of the ability range within a group. With respect to science education, no significant difference between heterogeneous and homogeneous grouping was found. It should be cautioned that the findings for science education rest on only four studies concerning physics and chemistry. Nevertheless, these studies did not agree on how to make up groups in order to maximize performance.
Lou et al. found an interaction between student’s ability level and group composition on achievement. Low-ability students profited most from being placed in groups with heterogeneous ability (d = 0.60), whereas medium-ability students benefitted more from being in homogeneous-ability groups (d = 0.51). These results have been replicated by more recent studies (e.g. [21] [3]).
The drawbacks found empirically for low- and medium-ability students can be interpreted in light of theory. In homogeneous low-ability groups, members are unable to help each other by providing information and giving support. According to Webb [19], medium-ability students in heterogeneous groups cannot profit from acting as tutors or tutees, since the high-ability students address themselves mainly to the members of low ability. Hence, medium-ability students should gain most from cooperating with their peers of the same ability by giving and receiving explanations.
For high-ability students the situation is less clear. Lou et al. did not find significant effects of group composition on performance (d = 0.09). However, the research results were very inconsistent. In elementary schools, Fuchs, Fuchs, Hamlett and Karns [22] composed homogeneous high-ability dyads as well as heterogeneous ones with one high-ability and one low-ability student. Video analyses revealed the superiority of homogeneous dyads for high-ability students. The interactions were more collaborative (e.g., better building on one another’s knowledge) and the handling of cognitive conflicts (e.g., success in resolving a cognitive conflict) was more effective. The mean differences were about one standard deviation. Hence, high-ability students in homogeneous dyads outperformed students of high ability in heterogeneous ones.
In line with this finding, Webb, Nemer, Chizhik and Sugrue [21] found advantages in placing students of above-average ability in homogeneous groups. In a follow-up study, Webb, Nemer and Zuniga [23] focused on the interaction within groups. High-ability students working in homogeneous groups uniformly performed well, and those in some heterogeneous groups performed as well as the high-ability students in homogeneous groups. That is, the outcome for high-ability students does not depend crucially on the composition of the group but strongly on the quality of the interaction (e.g., the quality of group discussion; positive socio-emotional behaviour). Interaction within a group predicted a student’s performance more strongly than did either the student’s ability or the overall ability of the group.
In order to promote active engagement by most or all group members, Webb [19] recommended homogeneous medium-ability groups, and groups with a moderate range of ability (that is, medium-ability students with those of high or low ability, respectively). Other types of groups (homogeneous high-ability groups, homogeneous low-ability groups, and heterogeneous groups made up of students with high, medium, and low abilities) might be detrimental to at least some students. In their research review, Neber, Finsterwald and Urban [24] found that high achievers should learn cooperatively in homogeneous groups with other high-achieving students.
1.3. Hypotheses
In this paper it is studied whether working in groups with homogeneous rather than heterogenous task-related prior knowledge can lead to positive outcomes for all students. As the meta-analysis conducted by Lou et al. [4] has shown, homogeneous grouping is often detrimental for low-ability students. In order to avoid this drawback, the learning environment implemented in our study is characterized as follows. First, in the jigsaw technique, teaching expectancy is established. This requires students to adopt the role of an expert, thus fostering interaction with their peers as well as with the learning material. Secondly, the learning material accommodates the needs of students who have different levels of prior knowledge of the topic being studied [25]. Written aids for performing the task as well as the answers to questions for repetition and comprehension were deposited on the teacher’s table. These aids were accessible to all students. They were encouraged to access this information in the case of significant gaps in their prior knowledge. It is hypothesized that homogeneous groups outperform heterogeneous groups. Furthermore, this should apply to homogeneous groups composed of students with high, medium as well as low prior topic knowledge.
2. Method
2.1. The Lessons
Our study was based on a learning unit that was developed by one of the researchers using the following criteria. For the jigsaw classroom it had to be possible to divide the material into independent segments, and their degree of difficulty had to be adapted to the studentscapabilities. Furthermore, the topic had to be meaningful that is, important for 12th-grade level as well as interesting. According to Häussler [26], boys as well as girls from 11 to 16 years of age have a higher than average interest in the structure of matter. Thus, gaining insight into the microworld by means of suitable equipment seems to be a favourite topic for very many students. Hence, it was suggested that the students should learn about the principles of the scanning electron microscope. This topic is particularly suitable for teaching physics at 12th-grade level, since the underlying physical principles (the motion of charged particles in electric and magnetic fields) cover a main area of the syllabus.
The following four sub-topics were discussed in the groups:
• The generation of the electron beam by acceleration of free electrons in the “electron gun
• The force on the electron beam due to the magnetic field of the coils (“Lorentz-force.
• The question, how the penetration depth into the sample depends on the energy of the electron beam and the atomic number of the sample material.
• The working principle of the electron detector (“Everhart-Thornley detector.
The students in the expert group were provided with written information about their sub-topic of the scanning electron microscope. In the expert groups, the students had to conduct an experiment in order to support the understanding of the respective physical principle and to foster interaction between the group members.
The learning unit as well as the tasks in the expert and jigsaw groups are described in more detail elsewhere [27].
2.2. Participants, Experimental Design, and Procedure
Twenty-two 12th-grade physics classes participated in the study. The analyses are based on 263 students without missing data. The students were instructed by their regular teachers.
The design of the study is shown in Figure 1. Some days before the experimental manipulation, students were pretested for prior topic knowledge.
The learning unit consisted of four school hours. First, basic information about the motion of electrons was introduced in two hours of direct instruction by the teachers. At the end of the first hour, the questionnaire concerning activation of deeper level processing strategies was given out as a pretest measurement. In the next two hours of physics (a double period), students worked in the jigsaw classroom. For cooperative working, the study topic was divided into four self-contained comprehensible sub-topics. Temporary groups of experts were formed, consisting of three to five students who were assigned the same sub-topic. They learned about their assigned sub-topic using prepared lesson materials and by conducting a suitable experiment. They were asked to study the material cooperatively in order to be prepared to teach it to their subsequent jigsaw group, in which each member had been assigned a different sub-topic. After working in the expert groups, the learning experience questionnaire was administered (activation of deeper level processing strategies; quality of communication). After the expert stage the students were assigned to the jigsaw groups randomly.
The post-test of academic performance was given in an extra lesson some days after the learning unit.
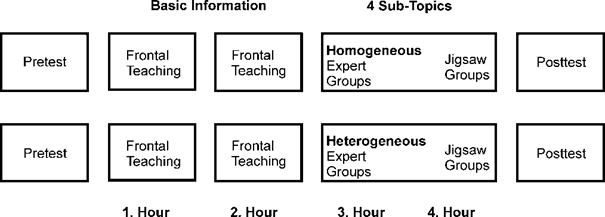
Fig. 1. Design of the study.
2.3. Independent Variable
In our study the effect of the composition of the expert group on different measures of outcome is experimentally investigated. The academic achievement in the pretest was used as the criterion for forming the expert groups. The pretest was designed to be highly topic related. According to the classification of Alexander, Schallert and Hare [28], the prior “topic knowledgeis assessed, that is, the specific knowledge that is relevant for understanding the physics of the electron microscope (e.g., knowing how to determine the force on moving electrons in a magnetic field in order to understand how the electron beam is scanned over the specimen). Following Renkl [29], prior topic knowledge should predict post-test performance better than unspecific knowledge of physics (“domain knowledge.
Based on the results of the pretest, the students were assigned to two grouping patterns:
• Students with similar scores for prior topic knowledge (homogeneous groups)
• Students with a broader range of scores for prior topic knowledge (heterogeneous groups)
Each of the participating classes was randomly assigned to one of these grouping patterns.
Homogeneous grouping. If the class was to be arranged homogeneously, the students with the highest pretest scores were put into expert group 1, working on the subtopic of electron guns. The students having the next highest scores were put into expert group 2 working on electron beam deflection and so on for expert groups 3 (working on penetration depth into the sample) and 4 (electron detector). So that subtopic 1 was not always assigned to the highest-performing students, in subsequent classes the four subtopics were randomly assigned to the expert groups 1.
Heterogeneous grouping. If the class was to be assigned to groups heterogeneously, the student with the highest pretest score was assigned to expert group 1. Then the student having the second-highest score was assigned to expert group 2, and students with ranks 3 and 4 to the expert groups 3 and 4, respectively. The next student was assigned to expert group 1, and so on.
In order to check the treatment the range of prior topic knowledge in homogeneous as well as in heterogeneous groups is assessed. The mean standard deviation in the homogeneous groups (7.4% of the maximum pretest score) was smaller than in the heterogeneous groups (18.1%). As expected due to the study design the difference is highly significant (t (75) = 6.4, p < .001).
2.4. Dependent Variables and Scale Analysis
2.4.1. Activation of Deeper Level Processing Strategies
Students responded to all questionnaires using a five-point scale in which only the first and fifth point were anchored (“not at all true” to “completely true”).
The items had to be constructed in a way that they were applicable for different learning situations (direct instruction and cooperative learning). The short scale proposed by Hänze and Berger [30] was used. The scale is given in the appendix. Cronbach’s alpha coefficient for the activation of deeper level processing strategies scale was .64.
2.4.2. Quality of Communication
The 8-item-scale called“quality of communication” describes the content-related aspects of cooperation as well as the social climate within the groups (see appendix). The items are based on a scale proposed by Jürgen-Lohmann, Borsch and Giesen [31], who referred to Johnson and Johnsonsbasic collaborative skills [32]. Items that were suitable for both the expert groups and the jigsaw groups were adopted and adapted. Cronbach’s alpha coefficient for quality of communication was .65.
2.4.3. Academic Performance
Students took pre- and post-tests in physics. These achievement tests were different and were constructed by the researchers.
The pretest encompasses four open items that assessed studentsprior topic knowledge of concepts that were essential for understanding the physics of the electron microscope in the jigsaw classroom. One example item is “Explain by a drawing how electrons in an electron tube are released and accelerated.Depending on how extensive the items are they were scored with 6 or 8 points, respectively.
The post-test covered studentsknowledge on the four sub-topics. This knowledge they had gathered either in the expert or the jigsaw groups. Each of the four sub-topics was tested by one open question. One example item is “Explain by a drawing how the electron detector in an electron microscope works The items were scored with 6, 10 or 12 points, respectively.
Pretest as well as post-test were corrected by a student with Bachelor’s degree in physics. Each item was scored based on a sample solution. The student was trained by one of the researchers beforehand. The interrater reliability between student and researcher was tested on a sub-sample of 23 tests. The Intraclass-Correlation was .96.
3. Results
The mean pretest score on prior topic knowledge was 14.4 points (max. 28) with a standard deviation of 5.75. Based on the pretest results, students as having low prior topic knowledge (00 points; 31% of the sample), medium prior topic knowledge (117 points; 40%), and high prior topic knowledge (188 points; 29%) were classified. That is, students who were classified as having medium prior topic knowledge were within a range of approximately half a standard deviation above or below the mean. Table 1 gives the mean scores in the pretest (in percent of max. score; standard deviation in brackets). As expected from the strategy that was employed for grouping, both compositions showed no significant difference in prior topic knowledge (F (1, 175) < 1, p > .50). That is, the group compositions were well balanced with respect to prior topic knowledge.
Table 1. Mean scores in pretest on prior topic knowledge (in percent of max. score; standard deviation in brackets).
| Prior topic knowledge |
Group composition | Low | Medium | High |
Heterogeneous | 28.3 (8.30) | 53.4 (7.51) | 76.0 (9.19) |
Homogeneous | 26.1 (9.78) | 49.4 (6.60) | 76.3 (9.73) |
In all of the following analyses small groups were nested within group compositions.
3.1. Effects of the Experimental Variation
3.1.1. Academic Performance
Since the expert groups were composed experimentally, the analysis is focused on that part of the test that covered the segment of learning material assigned to the student (“expert topic. In order to compare the level of achievement with respect to different expert topics, the scores for each subtopic were z-standardized.
The effects of group composition were analyzed by a univariate analysis of covariance with prior topic knowledge (high/medium/low) and composition of the expert group (homogeneous/heterogeneous) as factors. Means and standard deviations of academic performance on expert topics are depicted in Table 2.
Table 2. Adjusted means of academic performance in the post-test on expert topics (z-standardized; standard deviation in brackets).
| Prior topic knowledge |
Group composition | Low | Medium | High |
Heterogeneous | -.372 (.877) | -.393 (.864) | -.001 (1.11) |
Homogeneous | -.186 (1.04) | .568 (.833) | 652 (.869) |
A significant main effect for group composition (F (1, 175) = 21.64, p < .001) with medium to large effect size (Cohen’s d = .63) and a marginally significant interaction between prior topic knowledge and the composition of the expert group (F (2, 175) = 2.78, p = .065) was revealed.
In order to break down this interaction, three univariate analyses of variance according to the category of prior topic knowledge (low/medium/high) were conducted. A small (d = .19) but non-significant advantage for homogeneous grouping was found when comparing students with low prior topic knowledge (F (1, 36) < 1, p = .38). A homogeneous expert group was very beneficial for students with both medium (d = 1.13; F (1, 41) = 30.2, p < .001) and high prior topic knowledge (d = .66; F (1, 33) = 6.70, p = .014).
3.1.2. Learning Experience
With regard to activation of deeper level processing strategies univariate analyses of covariance with prior topic knowledge and composition of the expert group as factors were run. The corresponding pretest measure was used as covariate. For quality of communication a univariate analysis of variance was conducted, since no covariate was available for this variable. Both self-report scales were administered after the students had worked in the expert groups. The calculation revealed main effects for activation of deeper level processing strategies (F (1, 174) = 4.29, p = .040, d = .26), and quality of communication (F (1, 175) = 9.92, p = .002, d = .40) in favour of homogeneous groups (Table 3). No significant interaction between prior knowledge and composition of the expert group was found for activation of deeper level processing strategies (F (2, 174) < 1, p = .42), and quality of communication (F (2, 175) < 1, p > .50). Since no interaction was found, the separate categories (low/medium/high) of prior topic knowledge were not analyzed.
Table 3. Results from the learning-experience questionnaire (means; standard deviation in brackets).
| Group composition |
Dependent variable | Homogeneous | Heterogeneous |
Activation of deeper-level processing strategies | 3.57 (.687) | 3.39 (.672) |
Quality of communication | 3.97 (.513) | 3.75 (.599) |
Note. For activation of deeper level processing strategies the pretest measure was used as covariate. For quality of communication no covariate was available.
3.2. Test for Mediation
According to Webb et al. [23], the quality of group interaction is a strong predictor for student’s performance. In order to test whether the quality of communication in the expert groups could be regarded as a mediator between treatment (homogeneous vs. heterogeneous groups) and achievement with respect to the expert segment, the proposal of Baron and Kenny [33] was adopted (cf. Figure 2).
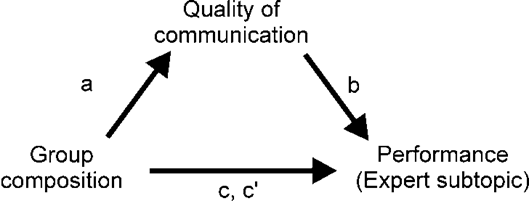
Fig. 2. Mediational links.
They recommended using a series of three regression equations (unstandardized regression coefficients, standard errors in brackets):
1. Expert performance is used as the criterion variable and homogeneity as the predictor: c = .621 (.110); p = .000
2. Quality of communication is used as the criterion variable and group composition as the predictor: a = .224 (.065); p = .001
3. Expert performance is used as the criterion variable and quality of communication as well as group composition as predictors: b = .242 (.097); p = .013 and c/i> = .570 (.111); p = .000.
In order to control for prior topic knowledge, the pretest scores were included as predictors in the analyses. The computation showed that, when controlling for the mediator, the unstandarized regression coefficient from topic to performance decreased from .621 to .570. Running the SOBEL-Test as proposed by Preacher and Hayes [34] showed that this decrease was statistically significant (z = 2.02, p = .043)2.
Following Baron and Kenny, the link from group composition over quality of communication to expert subtopic performance is thus a partial mediator. Activation of deeper level processing could not be identified as mediator.
4. Discussion
4.1. Overall Discussion
Regarding academic performance, in the present study it was found overall that students profit from working in groups that are homogenous with respect to prior topic knowledge. This general finding is in accordance with the meta-analysis conducted by Lou et al. [4]3. But, in contrast, the size of this effect is not small. Thus, the effect is highly relevant to the pedagogical settings. In order to assess this discrepancy one should bear in mind that not the intellectual capability as grouping criterion was used, but the prior topic knowledge. Students with low prior topic knowledge should be able to close their knowledge gaps with appropriate help from peers and appropriate learning material.
Why is it that students performed much better in homogeneous groups? In the present study a highly relevant composition criterion for learning success was introduced: The learning unit was essentially based on topic knowledge, accurately captured by the pretest measurement. That is, in homogeneous groups students shared a well-defined, common base of prior topic knowledge, which is highly relevant for successfully performing the task. Thus, we assume that new information might have been acquired more effectively. The activities could be carried out more smoothly, with fewer stops to help students who were not on a level with the rest of the group [35]. Thus, homogeneous groups may foster learning, as students are able to maintain a pace commensurate with that of other group members [4]. From a Vygotskian perspective, it might be inferred that the range of prior topic knowledge should be not too broad in order to involve all group members in fruitful discussions [36].
The better self-reported quality of communication within homogeneous groups was accompanied by the activation of deeper level processing strategies. Furthermore, quality of communication is a partial mediator from group composition to expert subtopic performance. According to De Fraine, Belfi and van Damme [37] these results are helpful to understand what happens in groups and how the group composition affects learning.
Regarding academic performance, a further analysis revealed that homogeneous grouping was favourable for all group compositions, although the effect was not significant for students with a low level of prior topic knowledge. Students with high and medium prior topic knowledge profited from homogeneity with medium to large effects, respectively.
In the meta-analysis of Lou et al. [4], low-ability students in particular profit from heterogeneous grouping. From a theoretical point of view this result might be explained by the help given to them by high-ability students. Why did students with low prior topic knowledge not suffer from missing such support in our study? We propose the following answer to this question.
The physics background was provided in previous lessons and should have been transferred to the new context of the electron microscope. The learning material was designed to support students with different levels of prior topic knowledge. As recommended by Gijlers and de Jong [36], additional support was offered (written information, placed on the teacher’s table), in order to compensate for gaps in prior topic knowledge. Furthermore, questions and answers for repetition and comprehension were offered in order to support the studentsunderstanding. Thus, it is hypothesized that this might have helped to compensate for the lack of helpful peers in homogeneous groups with low prior topic knowledge.
The significant differences from other studies on the effects of group composition may essentially be traced back to the cooperative learning technique that was adopted in the study: the jigsaw learning technique is inherently based on expert knowledge and teaching expectancy. From a role-theoretical perspective, enactment of a teaching role is unlikely if one’s self concept is not in agreement with the teaching requirement. This discrepancy might be highlighted when students cooperate with high-performing students in heterogeneous expert groups. This might be detrimental to self-esteem, associated with suboptimal learning results. This argument is in line with Buchs and Butera [38] who argue that working with a competent partner could be threatening to the student’s own competence and detrimental for learning when working on identical information as in the expert groups. The decision to seek help may be affected not only by the need for help, but also by the student’s judgement of the relative benefits and costs associated with seeking help [39]. A student with low prior topic knowledge may suffer a loss of perceived competence in his or her own eyes and in the eyes of the helper and significant others, especially when learning together with high-performing students in heterogeneous expert groups. Thus, with regard to seeking help, the learning outcome for students with low prior topic knowledge might suffer in heterogeneous expert groups.
4.2. Conclusions
In essence, the present study shows that students with medium and high prior topic knowledge profit from homogeneous grouping. No effect was found with respect to students with low prior knowledge. In order to support such students, the learning environment should encourage them to close their knowledge gaps, e.g., by offering written help. One should keep in mind that our study was based on a cooperative learning technique that structures the interaction within groups in a particular way, assigning “expertroles to students. Thus, the differences from other findings might in part be traced back to group dynamics as described by role theory. Furthermore, prior topic knowledge as the composition criterion is adopted, which is highly relevant for performing the task.
4.3. Limitations and Further Research
There are some limitations of this study. First, the students worked cooperatively for only two hours. Long-term cooperation might avoid some of the drawbacks of heterogeneous grouping. Furthermore, stable membership may enable students to develop a more cohesive group. Secondly, the study was conducted in advanced physics courses. Performance was partially determined by relevant prior knowledge and the higher the class, the greater its impact [40]. That is, in lower-level physics classes the beneficial effect of homogeneous grouping might be less distinct. Due to a lack of studies with participants in higher grades, especially at senior high-school level [24], further research is needed. Moreover, in the present study a self-report scale to assess communication within expert groups was used. Recording and analyzing the discussions in homogeneous and heterogeneous groups should shed further light on the interdependence of studentsverbal interactions (e.g., giving and receiving help [12]) and the learning success. With respect to role theory, one might test if the enactment of the expert role by low-ability students is constrained by the presence of high abilities in heterogeneous expert groups.
Appendix: Self-Report Scales
Activation of deeper level processing strategies
1. I felt focused.
2. I felt involved in learning the material.
3. I took a critical look at the new material.
4. I tried to distinguish between important and unimportant things.
5. I tried to connect what I was learning with things I already knew.
Quality of communication
1. I didn’t learn much in my group.
2. In my group I learnt how to handle topic-related difficulties.
3. Usually someone asked for details if something was difficult to understand so that everyone could reach a better understanding.
4. Every single member of our group contributed to the joint learning.
5. In our group we spent too much time discussing non-essential topics.
6. I disliked some group members so much that I didn’t even want to work with them on the assignment.
7. In my group I often didn’t ask a question if I didn't understand something.
8. The discussions in our group helped me to understand the topics better.
The values of items 1, 5, 6, and 7 were inverted before being summed. The mean of all items was used as an index for quality of communication.
Acknowledgements
The current research was supported by a grant from the Deutsche Forschungsgemeinschaft (DFG) allocated to both authors.
References
- Slavin, R. E., Hurley, E. A., & Chamberlain, A. (2003). Cooperative learning and achievement: Theory and research. In W. M. Reynolds, G. E. Miller, & I. B. Weiner (Eds.), Handbook of Psychology, Volume 7, Educational Psychology (Chapter 9, pp. 177 97). New York: Wiley.
- Lou, Y., Abrami, P. C., & Spence, J. C. (2000). Effects of within-class grouping on student achievement: An exploratory model. The Journal of Educational Research, 94 (2), 101-112.
- Saleh, M., Lazonder, A. W., & de Jong, T. (2005). Effects of within-class ability grouping on social interaction, achievement, and motivation. Instructional Science, 33, 105-119.
- Lou, Y., Abrami, P. C., Spence, J. C., Poulsen, C., Chambers, B., & d扐pollonia, S. (1996). Within-class grouping: A meta-analysis. Review of Educational Research, 66, 423-458.
- Aronson, E., Blaney, N., Stephin, C., Sikes, J., & Snapp, M. (1978). The jigsaw classroom. Beverly Hills, CA: Sage Publishing Company.
- Aronson, E. (2002). Building empathy, compassion, and achievement in the jigsaw classroom. In J. Aronson (Ed.), Improving academic achievement. Impact of psychological factors on education (pp. 209-225). San Diego, CA: Academic Press.
- Antil, L. R., Jenkins, J. R., Wayne, S. K., & Vadasy, P. F. (1998). Cooperative learning: Prevalence, conceptualizations, and the relation between research and practice. American Educational Research Journal, 35, 419-454.
- Bierman, K. L., & Furman, W. (1981). Effects of role and assignment rationale on attitudes formed during peer tutoring. Journal of Educational Psychology, 73, 33-40.
- Robinson, D. R., Schofield, J. W., & Steers-Wentzell, K. L. (2005). Peer and cross-age tutoring in math: Outcomes and their design implications. Educational Psychology Review, 17, 327-362.
- Allen, V. L. (1983). Impact of the role of tutor on behavior and self-perceptions. In J. M. Levine (Ed.), Teacher and student perceptions: Implications for learning (pp. 367-389). Hillsdale, NJ: Erlbaum.
- Renkl, A. (1995). Learning for later teaching: An exploration of mediational links between teaching expectancy and learning results. Learning and Instruction, 5, 21-36.
- King, A. (1999). Discourse patterns for mediating peer learning. In A. M. O’Donnell, & A. King (Eds.), Cognitive perspectives on peer learning (Chapter 4, pp. 87-115). Mahwah, NJ: Lawrence Erlbaum Associates.
- Webb, N. M., & Mastergeorge, A. (2003). Promoting effective helping behavior in peer-directed groups. International Journal of Educational Research, 39, 73-97.
- Kaartinen, S., & Kumpulainen, K. (2002). Collaborative inquiry and the construction of explanations in the learning of science. Learning and Instruction, 12, 189-212.
- Vygotsky, L. S. (1978). Mind in society: The development of higher psychological processes. Cambridge, MA: Harvard University Press.
- Slavin, R. E. (1996). Research on cooperative learning and achievement: What we know, what we need to know. Contemporary Educational Psychology, 21, 439.
- Dolmans, D. H. J. M., & Schmidt, H. G. (2006). What do we know about cognitive and motivational effects of small group tutorials in problem-based learning? Advances in Health Sciences Education, 11, 32136.
- Springer, L., Stanne, M. E., & Donovan, S. S. (1999). Effects of small-group learning on undergraduates in science, mathematics, engineering, and technology: A meta-analysis. Review of Educational Research, 69, 21-51.
- Webb, N. M. (1991). Task-related verbal interaction and mathematics learning in small groups. Journal of Research in Mathematics Education, 22, 366-389.
- Cohen, E. G. (1994). Restructuring the classroom: Conditions for productive small groups. Review of Educational Research, 64, 1-35.
- Webb, N. M., Nemer, K. M., Chizhik, A. W., & Sugrue, B. (1998). Equity issues in collaborative group assessment: Group composition and performance. American Educational Research Journal, 35, 607-651.
- Fuchs, L. S., Fuchs, D., Hamlett, C. L., & Karns, K. (1998). High-achieving studentsinteractions and performance on complex mathematical tasks as a function of homogeneous and heterogeneous pairings. American Educational Research Journal, 35, 227-267.
- Webb, N. M., Nemer, K. M., & Zuniga, S. (2002). Short circuits or superconductors? Effects of group composition on high-achieving studentsscience assessment performance. American Educational Research Journal, 39, 943-989.
- Neber, H., Finsterwald, M., & Urban, N. (2001). Cooperative learning with gifted and high-achieving students: A review and meta-analyses of 12 studies. High Ability Studies, 12, 199-214.
- Wilkinson, I. A. G., & Fung, I. Y. Y. (2002). Small-group composition and peer effects. International Journal of Educational Research, 37, 425-447.
- Häussler, P. (1987). Measuring studentsinterest in physics design and results of a cross-sectional study in the Federal Republic of Germany.International Journal of Science Education, 9, 79-92.
- Berger, R. & Hänze, M. (2009). Comparison of two small group learning methods in 12th grade physics classes focusing on intrinsic motivation and academic performance.International Journal of Science Education, 31,1511-1527.
- Alexander, P. A., Schallert, D. L., & Hare, V. C. (1991). Coming to terms: How researchers in learning and literacy talk about knowledge. Review of Educational Research, 61, 315-343.
- Renkl, A. (1997). Learning from worked-out examples: A study on individual differences. Cognitive Science, 21, 1-29.
- Hänze, M. & Berger, R. (2007). Cooperative learning, motivational effects and student characteristics: An experimental study comparing cooperative learning and direct instruction in 12th grade physics classes. Learning and Instruction, 17, 29-41.
- Jürgen-Lohmann, J., Borsch, F., & Giesen, H. (2001). Kooperatives Lernen an der Hochschule: Evaluation des Gruppenpuzzles in Seminaren der Pädagogischen Psychologie [Cooperative learning at the university: An evaluation of jigsaw in classes of educational psychology].Zeitschrift für Pädagogische Psychologie, 15, 74-84.
- Johnson, D. W., & Johnson, R. T. (1987). Learning together and alone. Englewood Cliffs, N J: Prentice-Hall.
- Baron, R. M., & Kenny, D. A. (1986). The moderator–ediator variable distinction in social psychology research: conceptual, strategic, and statistical considerations. Journal of Personality and Social Psychology, 51, 1173-1182.
- Preacher, K. J., & Hayes, A. F. (2004). SPSS and SAS procedures for estimating indirect effects in simple mediation models. Behavior Research Methods, Instruments, & Computers, 36, 717-731.
- Lawrenz, F., & Munch, T. W. (1984). The effect of grouping of laboratory students on selected educational outcomes. Journal of Research in Science Teaching, 21, 699-708.
- Gijlers, H., & de Jong, T. (2005). The relation between prior knowledge and studentscollaborative discovery learning processes. Journal of Research in Science Teaching, 42, 264-282.
- De Fraine, B., Belfi, B., & van Damme, J. (2011). Composition of learning groups. In N. M. Seel (Ed.), Encyclopedia of the Sciences of Learning (pp. 688-690). New York: Springer.
- Buchs, C., & Butera, F. (2009). Is a partner’s competence threatening during dyadic cooperative work? It depends on resource interdependence. European Journal of Psychology of Education, 24, 145-154.
- Nelson-Le Gall, S. (1981). Help-seeking: An understudied problem-solving skill in children. Developmental Review, 1, 224-246.
- Weinert, F. E., & Helmke, A. (1998). The neglected role of individual differences in theoretical models of cognitive development. Learning and Instruction, 8, 309-323.